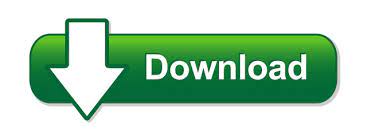
Proceedings of Machine Learning Research, Vol. In 29th Annual Conference on Learning Theory, V. Shamir (2016) The power of depth for feedforward neural networks. In Proceedings of the 2019 Conference of the North American Chapter of the Association for Computational Linguistics: Human Language Technologies, Volume 1 (Long and Short Papers), Toutanova (2019) BERT: pre-training of deep bidirectional transformers for language understanding. Proceedings of the 2018 Conference on Empirical Methods in Natural Language Processing, Training deeper neural machine translation models with transparent attention.
EUTRON TRANSFORMER CODE
Hongfei Xu and Josef van Genabith are supported by the German Federal Ministry of Education and Research (BMBF) under the funding code 01IW17001 (Deeplee). 61861130364), the Natural Science Foundation of Tianjin (Grant No. Deyi Xiong is supported by the National Natural Science Foundation of China (Grant No. Hongfei Xu acknowledges the support of China Scholarship Council (3101, 201807040056). xu2020lipschitz propose the Lipschitz constrained parameter initialization approach to reduce the standard deviation of layer normalization inputs and to ensure the convergence of deep Transformers. zhang2019improving propose the layer-wise Depth-Scaled Initialization (DS-Init) approach, which decreases parameter variance at the initialization stage, and reduces output variance of residual connections so as to ease gradient back-propagation through normalization layers. More recently, wei2020multiscale let each decoder layer attend the corresponding encoder layer of the same depth and introduce a depth-wise GRU to additionally aggregate outputs of all encoder layers for the top decoder layer, but residual connections are still kept in their approach. wu2019depth propose an effective two-stage approach which incrementally increases the depth of the encoder and the decoder of the Transformer Big model by freezing both parameters and the encoder-decoder attention computation of pre-trained shallow layers. wang2019learning propose the Dynamic Linear Combination of Layers (DLCL) approach which additionally aggregate previous layers’ outputs for each encoder layer. Table 7: Per-Layer Performance Reduction of the 6-Layer Transformer Base with Depth-Wise LSTM.īapna2018training propose the Transparent Attention (TA) mechanism which improves gradient flow during back propagation by allowing each decoder layer to attend weighted combinations of all encoder layer outputs, instead of just the top encoder layer. Table 6: Per-Layer Performance Reduction of the 6-Layer Transformer Base. While for the En-De task, the 12-layer Transformer with depth-wise LSTM may already provide both sufficient complexity and capability for the data set. We conjecture that probably because the data set of the Cs-En task ( ∼ 10 M) is larger than that of the En-De task ( ∼ 4.5 M), and increasing the depth of the model for the Cs-En task also increasing its number of parameters and capability. Unlike the En-De task, increasing depth over the 12-layer Transformer can still bring some BLEU improvements, and the 18-layer model results in the best performance. On the Cs-En task, the 12-layer model with our approach performs comparably to the 24-layer model with residual connections. Our analysis results support the moreĮfficient use of per-layer non-linearity with depth-wise LSTM than with The trained model into a linear transformation and observing the performanceĭegradation with the replacement. Layer's non-linearity on the performance by distilling the analyzing layer of Additionally, we propose to measure the impacts of the Our approach can bring about significant BLEU improvements in both WMT 14Įnglish-German and English-French tasks, and our deep Transformer experimentĭemonstrates the effectiveness of the depth-wise LSTM on the convergence ofĭeep Transformers. Our experiment with the 6-layer Transformer shows that
EUTRON TRANSFORMER HOW TO
LSTM for the Transformer, which shows how to utilize the depth-wise LSTM like Multi-head attention networks and feed-forward networks with the depth-wise

Relationship, and its design may alleviate some drawbacks of residualĬonnections while ensuring the convergence.

Networks applied to long sequences, while LSTM (Hochreiter and Schmidhuber,ġ997) has been proven of good capability in capturing long-distance Vanishing gradient problem suffered by deep networks is the same as recurrent
EUTRON TRANSFORMER SERIES
In time series instead of residual connections, under the motivation that the Transformers with the depth-wise LSTM which regards outputs of layers as steps We suggest that the residual connection has its drawbacks, and propose to train Model employs the residual connection to ensure its convergence. Increasing the depth of models allows neural models to model complicatedįunctions but may also lead to optimization issues.
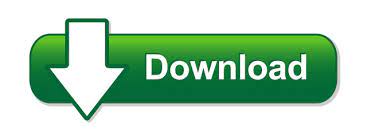